Models¶
I can never satisfy myself until I can make a mechanical model of a thing. If I can make a mechanical model, I can understand it. As long as I cannot make a mechanical model all the way through I cannot understand.
—Lord Kelvin
Introduction¶
Language echoes through the innermost reaches of the corners, so I will have the opportunity to mention most neural parcels, at least in passing. This breadth is very different from much of neuroscience, which tends to specialize in ever smaller brain bits. The danger of such a narrow focus has prompted a counter-trend, dubbed integrative neuroscience. Exactly what this means varies from researcher to researcher, but the journal Frontiers in Integrative Neuroscience asserts that it publishes research that “synthesizes multiple facets of brain structure and function, to better understand how multiple diverse functions are integrated to produce complex behaviors”. I’ll leave it up to you to decide whether this clarification makes you any wiser about whether to submit an article to the journal or not, because I want to present the topic inductively, not deductively.
My quandary is that I don’t know how much you know about language or neuroscience. In the absence of such shared knowledge, I don’t know exactly where to begin. I will thus fall back to what I hope is a common denominator, namely people’s behavior in general. Or at least people with psychiatric disease.
The organization of language in the brain¶
The traditional model¶
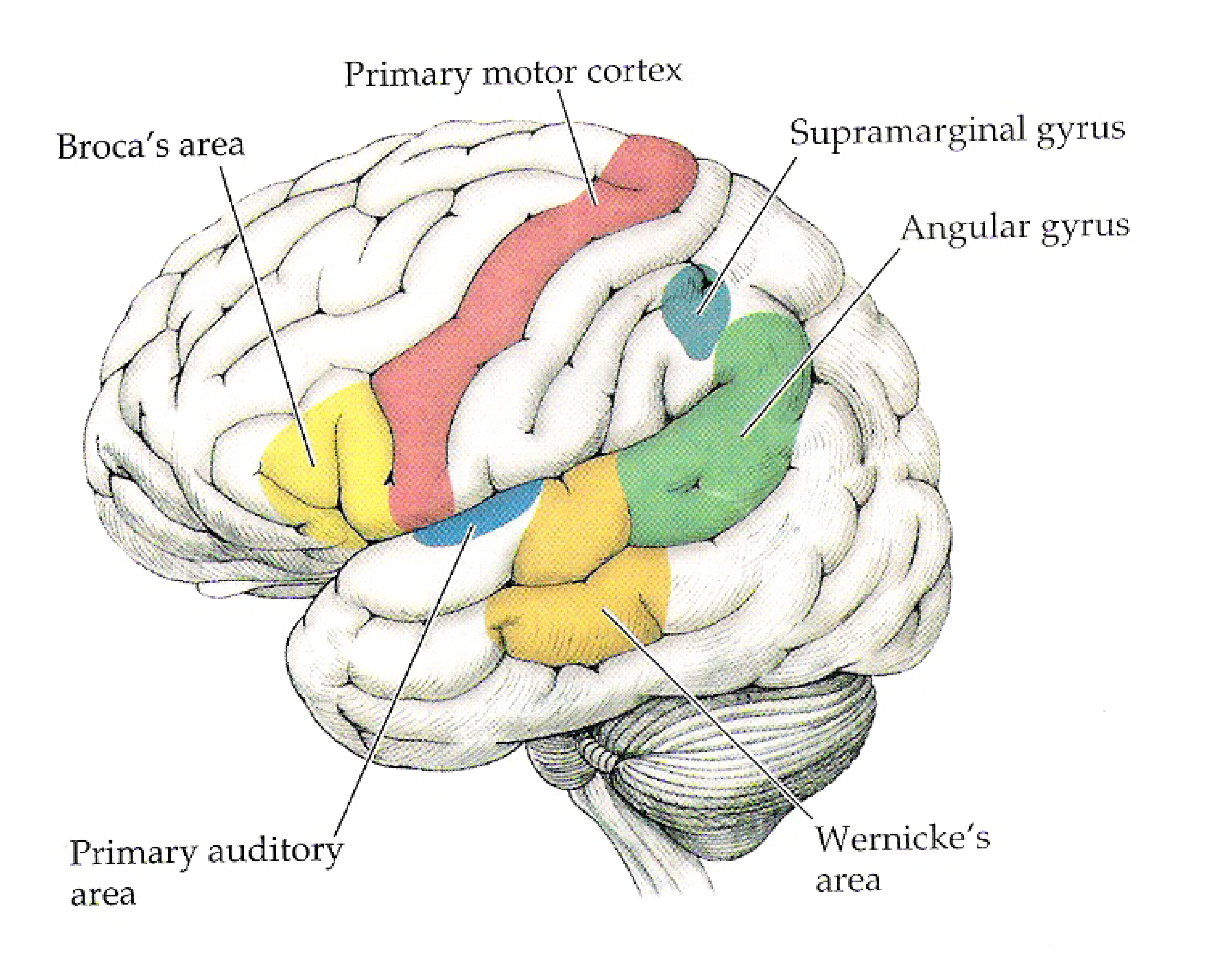
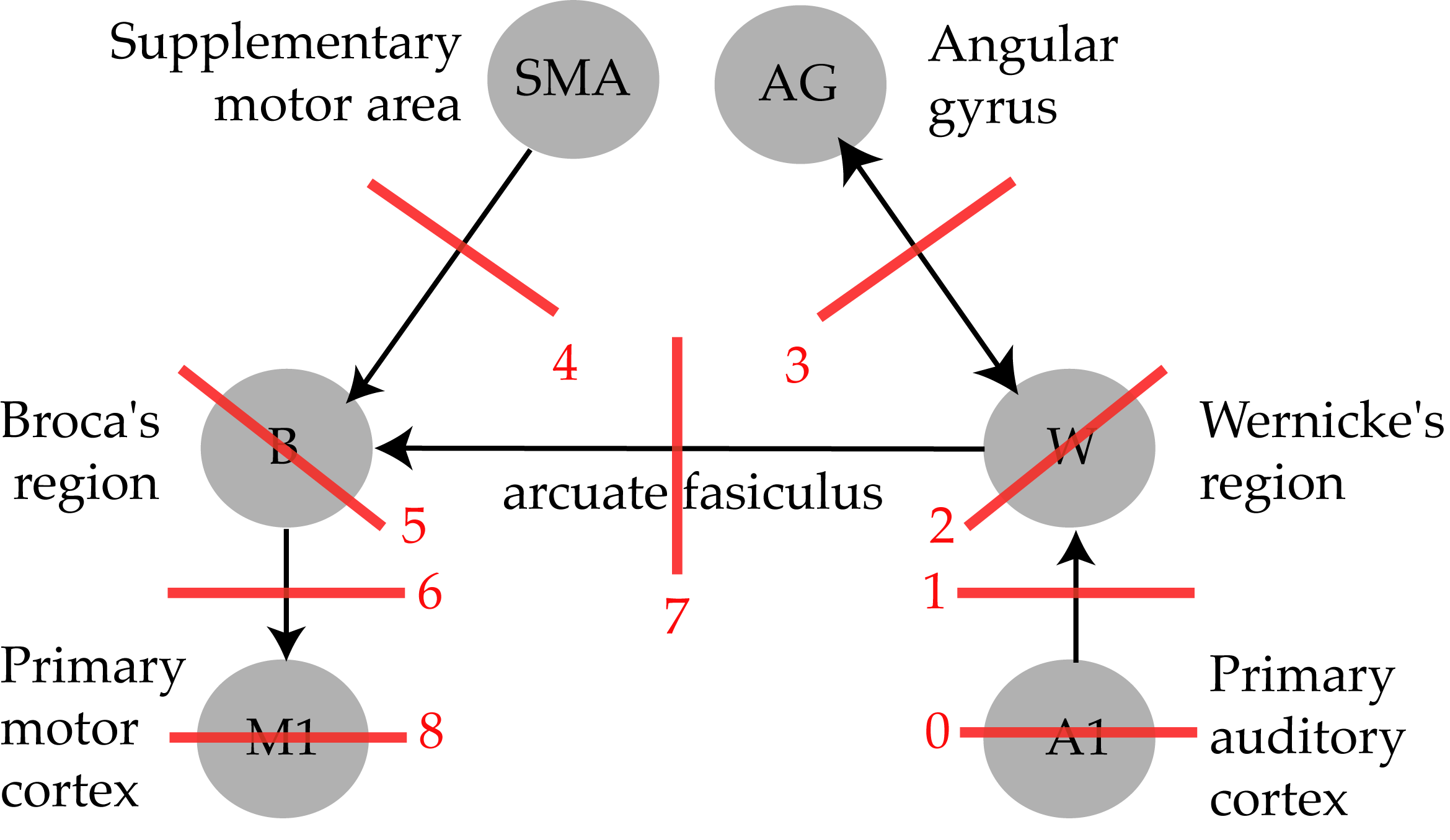
The Hickok-Poeppel model¶
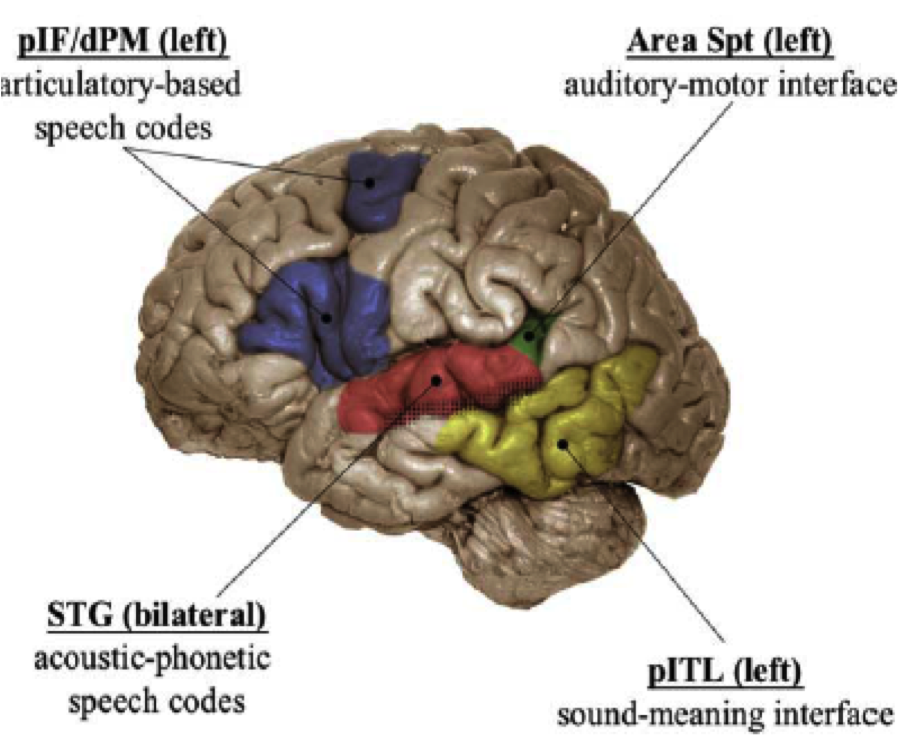
Fig. 81 Hickok & Poeppel (2004)’s model :cite:`HickokPoeppel2004` superimposed on the brain¶
Source: Copied from article.
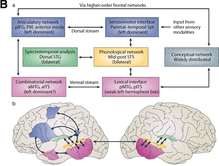
Fig. 82 Poeppel, Emmorey, Hickok and Pylkkänen (2014)’s model :cite:`PEHP12` superimposed on the brain¶
Source: Copied from article.
Tracts¶
https://commons.wikimedia.org/wiki/File:DTI_Brain_Tractographic_Image_A_panal.jpg
Akaishi’s psychiatric structured model¶
Tetsuya Akaishi (2018) has recently proposed an integrative framework for psychiatric disease, such as attention-deficit/hyperactivity disorder, autism spectrum disorder, obsessive compulsive disorder, post-traumatic stress disorder, and schizophrenia. The framework consists of three loops.
The input-output-feedback loop¶
This first and most basic loop connects the internal and external worlds. By “external world” we mean the realm of the senses, but Akaishi’s psychiatric bent leads him to highlight specific sorts of sense data such as the task to be performed or feedback from the performance of previous tasks – which are really implicit in the incoming sensory data and so should not be isolated from it. The “internal world” takes this sensory input, filters and processes it via a module of perception and then submits it to a module of personality (sense of values) and intelligence (reward anticipation), upon which memory acts. This internal world can produce an output, an action, that is broadcast to the external world of the social and family environment, resulting in some more sensory data that impinges on the entrance of the internal world, creating a feedback loop.
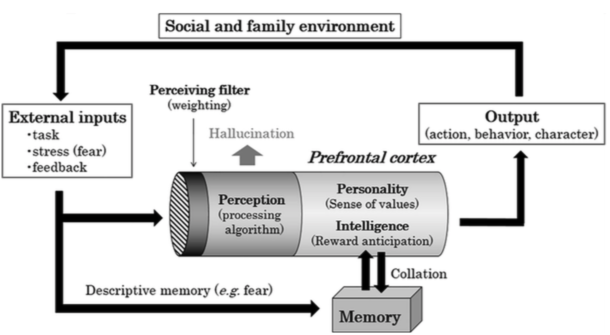
Fig. 83 The input-output-feedback loop¶
Fig. 1 from Akaishi (2018).
The main application of the input-output-feedback loop to psychiatric disease is that a mis-function in perception leads to hallucination.
More interesting to us is to speculate on how language would use this loop. Imagine that the environment of the external world produces the linguistic utterance “What is your name?”. It qualifies as an input to the internal world, which is filtered into something like ‘linguistic auditory data’, perceived as the speech [] and converted into the meaning of the question, to generate the answer action of articulating “Harry”, an output back to the external world.
The perception-action loop¶
We can be much more precise.
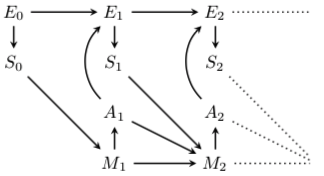
Fig. 84 First two time steps in a perception-action loop, where E is the environment, S is a sensor value, M is the memory, A is an action, and the numerical subscripts indicate times.¶
From {Biehl et al., 2018, CT}, Fig. 1.
The diagram is read from left to right, beginning at time 0, in which the environment is in state \(E_0\) which is perceived as \(S_0\). Then the clock ticks once, and several things happen. The sensory reading \(S_0\) is put into memory, \(M_1\), which triggers the first action, \(A_1\), which becomes part of the next environmental state \(E_1\), which also contains input from the initial state \(E_0\). Finally, \(E_1\) is read by the sensor to produce \(S_1\).
The same sequence is repeated for tick 2 … and then indefinitely.
The only difference is that M keeps track of everything that has happened in the past. Thus \(M_2\) contains not only the previous memory \(M_1\) but also the previous action \(A_1\) and the previous sensor reading \(S_1\). Putting the ever-growing store of states and actions into a mathematical notation may help you to appreciate how the entire system works:
Notation
Practice
What would be the contents of \(M_3\)?
Make up specific states and the corresponding actions for times 0 to 3.
Policy¶
It will be useful to have a name for such a sequence of states and actions. Taking a cue from the literature on reinforcement learning, let us call it a policy.
Practice
I took a few usages of the word policy from Google News. Decompose them into a sequence of states and actions, if possible.
The reward-learning loop¶
The basic perception-action cycle does a lot, but we don’t know how. To remedy this problem Akaishi’s next step is to add a mechanism for evaluating how well a given action applies to a sensory state. Following a long tradition, Akaishi labels this evaluation reward. The idea is simple: if you are experiencing the sensory state of hunger, your cognitive system should reward the action “go for pizza” more than “play chess”. At least, I think it works that way for most people – you may find chess as satisfying as a good meal (so your reward system is different).
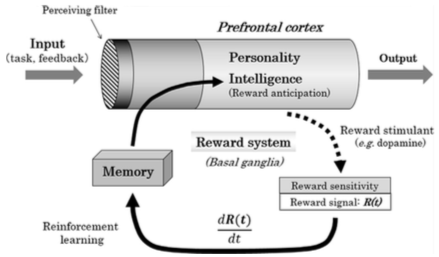
Fig. 85 The reward-learning loop¶
From Akaishi (2018), Fig. 2.
Let us try to translate this image into words, using the toy example embedded in an action-preception loop. Every time your cognitive system is faced with the sensation of hunger \(s_{hunger}\) and the alternative actions of getting some food \(a_{food}\) or playing chess, \(a_{chess}\), the preference for \(a_{food}\) should be strengthened. If we assign small numbers for the reward R(S,A) of either action at time 0, say 0.1, then the learning would take this form:
Notation
Misfunctioning of the reward system could be the root of depression. Akaishi suggests that it could arise from a very low setting of R() for all state-action pairings, even after learning.
Question
Explain in your own words what the result would be in a perception-action cycle.
A loss of interest in previously pleasurable activities is generally termed anhedonia.
The mood-reward sensitivity loop¶
The final component of Akaishi’s model is mood, which acts to weaken or strengthen the reward signal. The idea is that positive mood increases reward while negative mood decreases it.
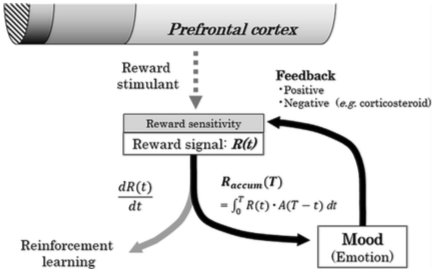
Fig. 86 The mood-reward sensitivity loop¶
From Akaishi (2018), Fig. 3.
Akaishi formalizes it as a multiplication of \(R(S,A)_T\). If multiplication of a number by 1 just returns the number, multiplication by a number greater than 1, say 1.1, returns a slightly larger number (amplification), while multiplication by a number less than 1, say 0.9, returns a slightly smaller number (attenuation). I am going to state this in a different way, as so:
Notation
(4) uses the term g for “gain” because, in signal-processing theory, a gain enhances (or attenuates) a signal in a noisy environment via a multiplication of the signal by the gain quantity.
Akaishi speculates that panic disorder and obsessive compulsive disorder derive from inaccurate anticipation based on irregular inputs of fear-related memories [(OCD) are also known to be frequently accompanied each other [85]. Although the timing and manifestation of these disorders are different, both of them , possibly caused by impaired inhibitory learning of the PFC [86,87].]
A more intricate resolution of the reward system could be found in schizophrenia. Akaishi relates that a first problem can be found in the perceptual system, since there are many reports of hallucination in schizophrenic patients. More relevant to our present concerns, they also betray a certain amount of anhedonia, which suggests that the background or tonic level \(R(S,A)_0\) is abnormally low. Paradoxically, they also show a heightened response to specific sensory states, suggesting that the learning level \(R(S,A)_{t > 0}\) is abnormally high.
In mood disorders, decreased amount of reward signals and elevated serum corticosteroid levels have been already confirmed [54–57]. In normal healthy population, such an elevated corticosteroid level would suppress further secretion of corticosteroid with a negative feedback, but this feedback system is known to be impaired in those with mood disorders [43]. As a result, elevated serum corticosteroid levels in mood disorders would further suppress the reward sensitivity and exacerbate the mood conditions [55–57]. This elevated serum corticosteroid, fol- lowed by suppressed reward sensitivity, also impairs the efficiency of reinforcement learning. As a result, memory consolidation, memory retrieval, and social learning are all going to be disturbed in mood disorders [58,59].
Inherent motivation¶
Inherent motivation¶
Empowerment¶
Summary¶
References¶
Akaishi, Tetsuya. “Unified Neural Structured Model: A New Diagnostic Tool in Primary Care Psychiatry.” Medical Hypotheses 118 (2018): 107-13.
Pezzulo, Giovanni, Francesco Rigoli, and Karl J. Friston. “Hierarchical Active Inference: A Theory of Motivated Control.” Trends in Cognitive Sciences 22.4 (2018): 294-306.
Biehl, Martin, Christian Guckelsberger et al. “Expanding the Active Inference Landscape: More Intrinsic Motivations in the Perception-Action Loop.” arXiv 1806.08083 (2018)
The next topic¶
The next topic is Frequency.
Last edited Aug 22, 2023